How to Understand & Calculate Statistical Significance [Example]
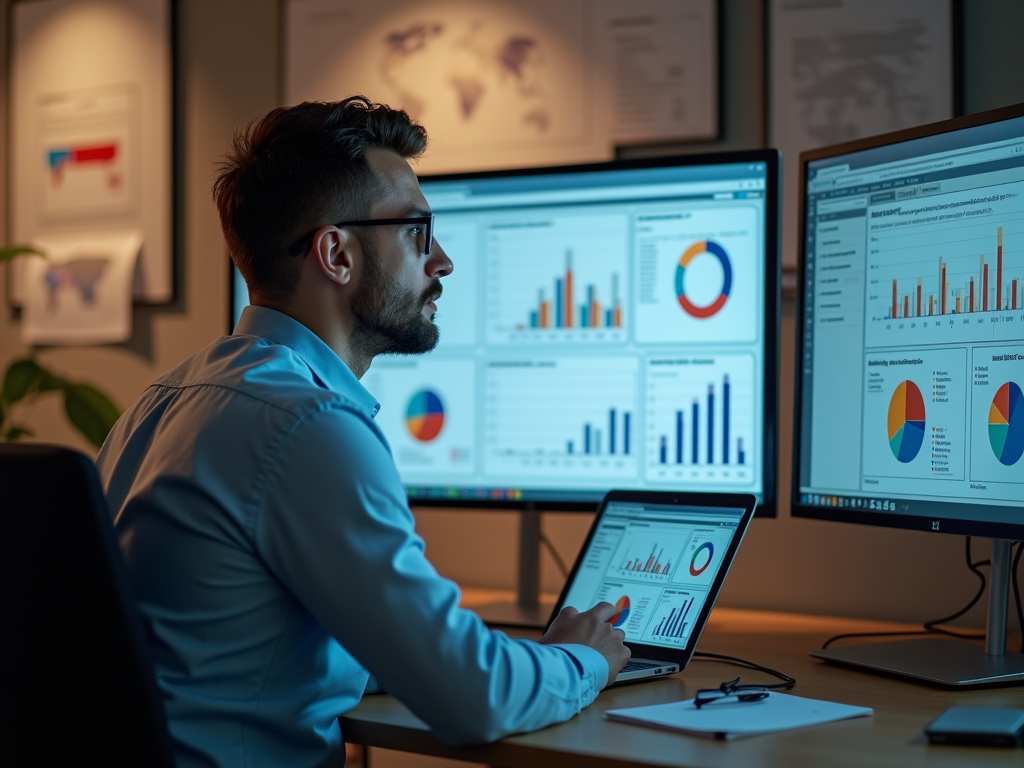
In a world increasingly driven by data, understanding statistical significance has become imperative for anyone looking to make informed decisions. Statistical significance serves as a critical pillar in interpreting research findings, ensuring that conclusions drawn from data aren’t just a product of random chance. Whether you’re a researcher analyzing your latest experiment, a marketer assessing campaign effectiveness, or a student diving into a complex data set, grasping the nuances of statistical significance will enhance your analytical prowess. We will take a deep dive into what constitutes statistical significance, how to calculate it, and provide a concrete example to solidify your understanding. By the end of this article, you will be equipped with essential knowledge that will empower you to navigate the complexities of data interpretation confidently.
Furthermore, we aim to demystify common concepts related to statistical significance, ranging from hypotheses to P-values. These fundamental ideas are not just academic jargon; they carry real-world implications that can make or break the validity of your conclusions. By mastering these concepts, you’ll position yourself to better evaluate research findings and contribute to discussions with greater confidence. This article will offer a structured approach for understanding statistical significance, making it accessible whether you are a novice or an experienced statistician.
What is Statistical Significance?
Statistical significance is a mathematical measure that helps determine if your results are likely due to chance or if they indicate a true effect. Understanding this concept is crucial for researchers, analysts, and anyone involved in data interpretation. Essentially, it indicates whether the observed data would be unusual if the null hypothesis were true. The goal becomes clear: to ascertain whether the effects observed in a study can be reliably attributed to the intervention being tested rather than random variability. When we embrace statistical significance, we open the door to making more informed decisions based on empirical evidence, leading to better outcomes in various fields.
Statistical significance plays a pivotal role in research by:
- Indicating the reliability of your results.
- Helping to make informed decisions based on data.
- Preventing misleading conclusions from random variation.
Key Concepts in Statistical Significance
Before diving into calculations, it’s essential to understand the fundamental concepts related to statistical significance. These concepts form the foundation upon which statistical tests are built. Understanding them will not only aid in your analysis but will also enhance your ability to communicate findings clearly. Among these concepts are the null and alternative hypotheses, the P-value, and the alpha level. Let’s explore these concepts a bit further to establish a strong grounding in statistical significance.
Null Hypothesis and Alternative Hypothesis
The two cornerstone hypotheses in statistics are:
- Null Hypothesis (H0): This is the default assumption that there is no effect or difference between groups.
- Alternative Hypothesis (H1): This states that there is an effect or a difference.
P-Value
The P-value is a crucial metric in statistical testing, representing the probability of obtaining the observed results if the null hypothesis is true. A lower P-value (typically < 0.05) suggests that the results are statistically significant. To make sense of P-values, consider them as reflective of how extreme your data is under the null hypothesis. As you dig deeper into your analysis, understanding the P-value’s implications will become ever more critical.
Alpha Level (α)
The alpha level is the threshold set before conducting a test, commonly at 0.05. If the P-value is lower than the alpha level, the results are considered significant. It’s important to note that the choice of alpha level may differ based on the context and field of study. This level acts as a gatekeeper, guiding researchers in their decision-making processes. Below is a table summarizing some common alpha levels and their applications:
Alpha Level | Common Use Cases |
---|---|
0.05 | Standard threshold for many social sciences |
0.01 | Used in more conservative research settings |
0.001 | Applied in highly critical scientific investigations |
How to Calculate Statistical Significance
Calculating statistical significance involves several steps, often requiring specific formulas and statistical tools. To make this process straightforward, we’ll outline key procedures below. Following these guidelines will help ensure that your analysis is both accurate and credible. While each step may vary depending on the chosen test, the overarching principles will remain consistent across different methodologies.
The following list summarizes the essential steps for calculating statistical significance:
- Define the Hypotheses: Clearly articulate your null and alternative hypotheses based on your research question or problem.
- Choose the Right Statistical Test: Select the appropriate statistical test based on your data type and distribution, such as:
- t-test
- Chi-square test
- ANOVA
- Collect and Analyze Data: Gather your data and perform the chosen statistical test using software tools or manual calculations.
- Calculate the P-Value: Use the results from your statistical test to find the P-value. Statistical software often provides this value automatically.
- Compare P-Value with Alpha Level: Assess whether the P-value obtained is less than the predetermined alpha level. If so, you can reject the null hypothesis.
Example of Calculating Statistical Significance
To illustrate the concept, we’ll go through a straightforward example involving a t-test. Understanding how to apply these principles to a real-world scenario will reinforce your learning and enhance your confidence in statistical analysis. Let’s explore an example where we want to determine if a new teaching method is more effective than the traditional method.
Scenario
Suppose we want to determine if a new teaching method is more effective than the traditional method. In this example, we have two groups of students being taught using different methodologies. This setup will allow us to compare average performances directly. Below is a summary of the data:
Group | Teaching Method | Mean Score | Sample Size (N) |
---|---|---|---|
Group A | Traditional Method | 75 | 30 |
Group B | New Method | 85 | 30 |
Calculation Steps
Here are the steps to calculate the statistical significance in this example:
- State the Hypotheses:
- H0: There is no difference in mean scores.
- H1: The new method results in a higher mean score.
- Conduct the t-test: Perform the calculation or use statistical software to find the t-value and P-value.
- Interpret Results: Assume we calculate a P-value of 0.03.
- Conclusion: Since 0.03 < 0.05, we reject the null hypothesis and conclude that the new method is statistically significant.
Conclusion
Understanding and calculating statistical significance is essential for validating research findings. By leveraging key concepts like hypotheses, P-values, and statistical tests, you can confidently assess whether your results are meaningful or due to chance. The principles outlined in this article can empower you in your research and decision-making processes, allowing you to derive actionable insights from data analysis. In this age of data-driven decision-making, mastering statistical significance will not only enhance your research capabilities but also enable you to engage with data in a meaningful way. Embrace these tools, and watch as your confidence in interpreting data continues to grow.
Frequently Asked Questions
- What does a P-value of 0.05 mean? A P-value of 0.05 indicates there is a 5% probability that the results occurred by chance, suggesting statistical significance.
- How do I choose the right statistical test? The choice depends on the data type (e.g., categorical vs. continuous), number of groups, and whether the data meets the assumptions of the test.
- Can statistical significance guarantee practical significance? No, statistical significance does not imply that the results are practically important or relevant in real-world applications.
- What is a Type I error? A Type I error occurs when the null hypothesis is incorrectly rejected, indicating a false positive.
- Is a smaller P-value always better? While smaller P-values suggest stronger evidence against the null hypothesis, they must be interpreted in the context of the study and research question.